Welcome to the world of AI, where technology is changing things in amazing ways. In this world, there’s a big challenge: how can we train AI models together without giving away our secrets? That’s where Federated Learning comes in.
It’s like a special tool that lets us work together on AI training while keeping our private stuff safe. Normally, when we train AI models, we put all our data in one big place. But that can be risky because our personal info might not be safe there. Federated Learning fixes this by spreading the training process out to different devices like phones and computers. Each device learns a bit using its own data, but it doesn’t share any private info. It’s like teamwork for AI, where everyone helps without sharing secrets. With Federated Learning, we can make AI smarter while making sure our personal stuff stays private. Let’s dive into how it works and why it’s so cool! Welcome to the AI world where technology is transforming things in exciting ways! In this world of ours, a major problem arises; how can we learn artificial intelligence models at once without leaking our secrets? This is what Federated Learning does for us. It’s like a magical tool that enables cooperative training on AI while keeping our private things.
Normally, we normally put all our data together in one place when we’re training AI models. However, because of taking such a step it would be dangerous since some personal info may not be secure there. This problem is solved by Federated Learning through distributing the learning process to various devices like mobiles and computers. Each device learns slightly with its own information, but it doesn’t mix its private information with anyone else’s. It’s like teamwork for AI, where everyone helps without sharing secrets. We could make AI smarter and keep our personal stuff secret with Federated Learning. Let us dive into how this thing works and why it is so nice!
“Federated Learning is like teamwork for AI. We can work together on projects without giving away our secrets.” – Dr. Alice Zhang, AI Researcher
Understanding Federated Learning
Perhaps federated learning appears to be a complicated idea, but let’s simplify it. Suppose you are in a big project team and each individual has his or her set of notes. But instead of gathering together in one place so as to share all such material, everybody sits down with their notes and learns something new. In the era of AI, this is somewhat similar to how Federated Learning functions. It does not aggregate all data in one central point such as huge computer servers; rather, the work is distributed if not shared by Federated Learning. More specifically, every device (a smartphone, laptop or any other smart gadget) trains on its own dataset without necessarily being obliged to share everything with others. Then only these necessary updates –which are more or less small nuggets of information- are communicated back to the central server. In this manner your private data remains safe and secure while AI becomes smarter without having full knowledge about you.
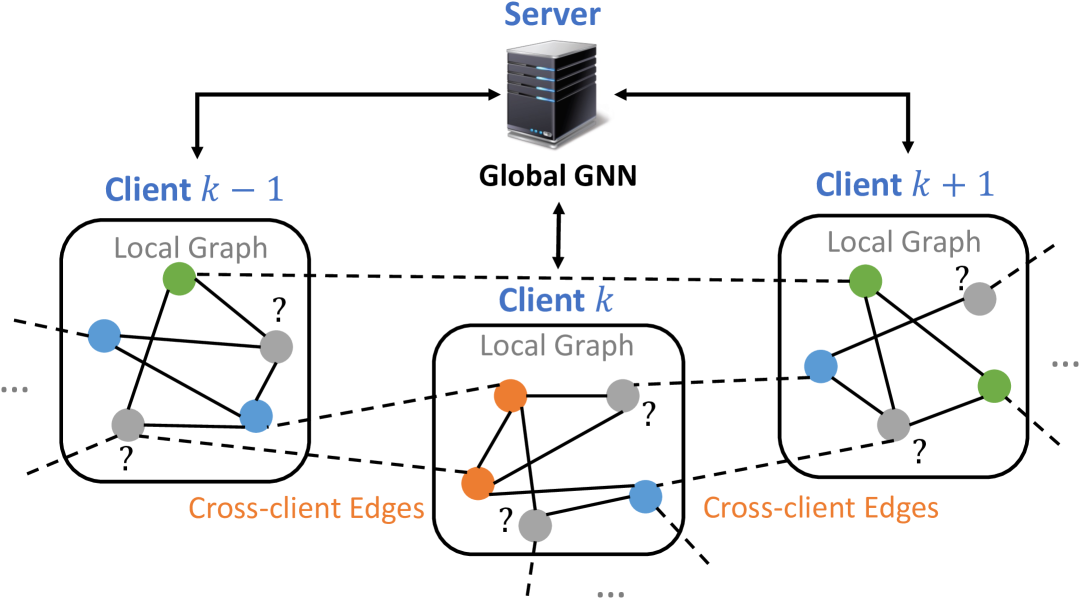
To sum up, federated learning enables us contribute towards AI training while ensuring privacy protection for ourselves. It is like acting collaboratively where we all perform our parts and finally gain from better AI without losing our personal privacy.
Advantages of Federated Learning:
- Privacy Protection: The core of Federated Learning lies in keeping the sensitive data away from centralized database openly accessible after being collected by individual devices. Such decentralized system does not allow data breaches and unauthorized entry to sensitive data hence it is a perfectly suitable solution for privacy and security of users.
- Scalability: Federated Learning is able to cope up with the volume of deployments and end-point networks in a big size without compromising its performance. Working on the distribution of the training operation to personal devices, the Federated Learning option makes possible to avoid central information processing and hence decrease communication load, as well as provide fast model training on AI.
- Edge Computing Integration: Federated Learning does this by combining AI model training and processing in so that edge computing infrastructure can power devices like smartphones, tablets, and IoT that can run and change (train) models at the edge. It leads to low delay, minimal use of bandwidth as well as favouring general decision-making by making Federated Learning appropriate for the Edge computation model.
- Cost-effectiveness: What happens to the prices of publicly handing the data storage and transmission is that they are divided into the dissemination of the learning process, which occurs on the individual devices. Blocking the data exchange and storage, Federated Learning creates only the tad bit of communication overhead and data transmission cost, and thus it is a much superior approach for economizing large-scale AI assimilation.
Fact: Federated Learning can reduce data sharing by up to 90%, keeping our info safe while making AI smarter.
Applications of Federated Learning:
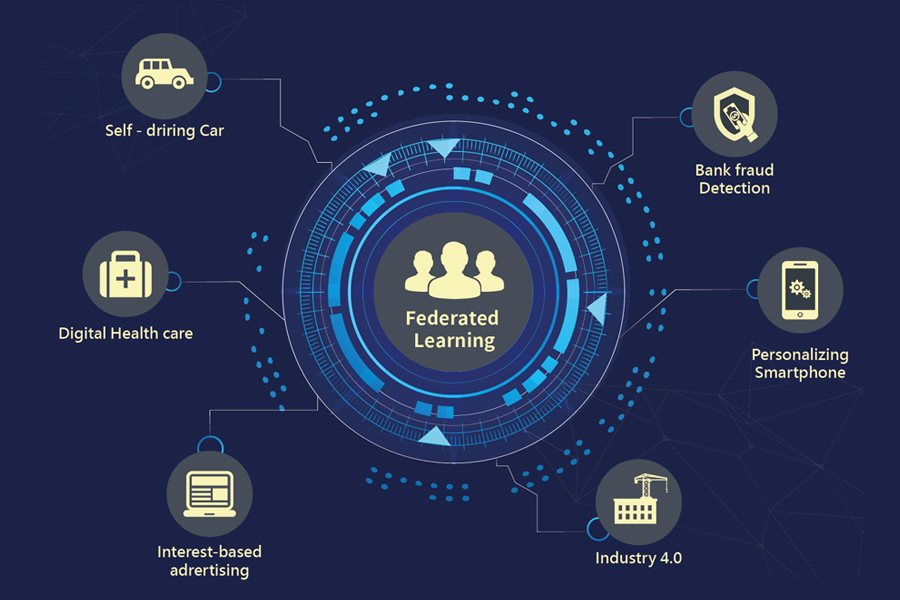
- Healthcare: Federated Learning helps doctors and researchers analyze medical data from different hospitals without sharing patient information. This allows for better disease diagnosis and treatment recommendations while keeping personal data private.
- Personalized Shopping Recommendations: Online shopping platforms use Federated Learning to suggest products based on your browsing history without accessing your personal data. This helps improve your shopping experience while protecting your privacy.
- Smartphones and Apps: Your smartphone uses Federated Learning to learn from your usage patterns and improve features like keyboard suggestions or voice recognition. This makes your device smarter without sharing your personal information with others.
- Traffic Prediction and Navigation: Navigation apps use Federated Learning to predict traffic patterns and suggest alternate routes without tracking your exact location. This helps you reach your destination faster while keeping your movements private.
- Language Translation: Language translation apps use Federated Learning to improve translation accuracy by learning from users’ interactions without accessing their private messages or conversations. This helps break down language barriers while ensuring privacy.
Challenges and Solutions
- Communication Challenges:
- Challenge: For F(L) to work, devices must be able to communicate with one another and the server. One of the biggest hurdles is that there aren’t always good connections, which might result to slowdowns.
- Solution: People are trying on developing approaching about how devices could talk more efficiently and it could be done much faster in Federated Learning.
- Data Differences:
- Challenge: These devices often have a lot of data, some of which may be incompatible, which makes it hard to train the model.
- Solution: The Model-Agnostic Meta Learning that is presented in the most recent version takes into account diverse data types and actually trains one particular device to help another carry on with training.
- Keeping Models in Sync:
- Challenge: Federated learning pursues the coordination of models from different devices by means of a shared work space as well as promotes the integration of data from all sources.
- Solution: There are options very similar to the approach used, syncing up models based on devices and the network in a hook to keep things running smoothly.
Impressive Fact: Did you know that Federated Learning can reduce data transmission by up to 90%? That means it’s super-efficient at sharing information between devices without needing to send all the data every time. This not only saves time and resources but also helps keep your personal information safe and private. With Federated Learning, we can make AI smarter while protecting our privacy – now that’s impressive.
Future Directions:
However, I am certain that Federal Learning has a bright future in coming times because of the almost endless exciting prospects! The people are trying to improve it and create the environment, which allows many people to use it in various ways. They are working on the “Thing” in which interaction between devices is made much easier so that, not only can they share information faster, but they can also learn from each other through efficiency. Besides that they also find out novel ways they can analyse a variety of data and protect it all from being misused. We can assure that our information is only for authorized use.
We are curious about how federated learning will be used so that in the future it might be possible that doctors can find new and better treatments sooner, using our phones more intelligently and improving how we can move around by car for instance. Increasing the research and innovation capacity, Federated Learning would continue its expansion and digital life upgrade. The information security issue, however, is still remaining. Hence, strap yourself in for a trip into Federated Learning that would be exciting.
Conclusion:
In the wide array of AI, Federated Learning distinguishes itself as a great invention that can solve one big problem; how to jointly train AI models without having to compromise our privacy. The thing is, it’s like having a superman in the form of an application that helps us work together on AI projects while protecting our personal data. Federated Learning enables all of us to function as a team thereby allowing us share knowledge but keep our secrets intact. The future of Federated Learning looks bright and promising. As technology advances, Federated Learning will be central in making AI even smarter and more useful. It means there is so much we can do with AI yet everything remains secure and private. Because of further research and enhanced developments, Federated Learning will continue to grow thereby making life easier for
people.
Let’s therefore embrace this exciting technology and its potential for a better future. Thanks to leading brands like Google’s Federated Learning, we are going towards such a time when artificial intelligence shall partner with humans against privacy intrusions instead of being the threat. It should be technology that leads us into not just shaping but also defining our own futures based on trust, transparency, and respect for personal information.
Additional Resources:
Books:
- “Federated Learning” by Qiang Yang, Morgan & Claypool
- “Advances and Open Problems in Federated Learning” by Peter Kairouz, Foundations and
Trends® in Machine Learning - “Federated AI for Real-World Business Scenarios” by Dinesh C. Verma, CRC Press
- “Federated Learning for IoT Applications” by Satya Prakash Yadav, Springer
- “Federated Learning for Wireless Networks” by Walid Saad, Springer
- “Automated Machine Learning and Federated Learning” by Andreas Deppler, Wiley
- “A Blockchain-Based Federated Federated Learning: Concepts and Applications” by Ankit
Khushal Baral, IGI Global
Online Courses:
- Coursera – “Advanced Deployment Scenarios with Tensorflow” by Stanford University
- Udemy – “Federated Learning Using PyTorch” by Mohammed Gharibi
- Udemy – ” Crypto Data Science and ML with Python” by Mammoth Interactive, John Bura
- Class Central – “Federated Hyperparameter Tuning – Challenges, Baselines, and
Connections” by Stanford MedAI
These resources offer valuable insights and educational opportunities to deepen your understanding of Federated Learning, privacy-preserving machine learning, ethical considerations in AI, and governance strategies for responsible AI deployment.